When

Monday, November 25th, 2024 - 12:00 p.m.
Courtney Comrie and Yisha Tang
PhD Candidates
Biomedical Engineering
University of Arizona
Keating 103
Zoom link | Password: BearDown
Hosts: Alex McGhee and Swarna Ganesh
(Instructor permission required for enrolled students to attend via Zoom)
Persons with a disability may request a reasonable accommodation by contacting the Disability Resource Center at 621-3268 (V/TTY).
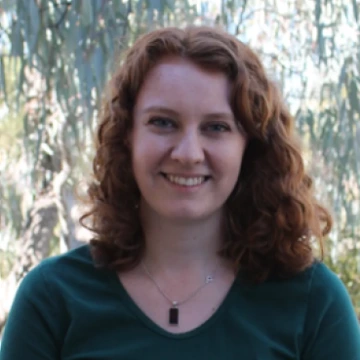
Courtney Comrie
Hutchinson Lab
"Comparisons in Advanced Diffusion Techniques in Phantoms and Alzheimer’s Disease"
Abstract: Alzheimer’s disease (AD) is an irreversible degenerative brain disease affecting 6.7 million Americans, with hallmark pathologies of plaques and tangles. However, clinical markers for early diagnosis are lacking, and up to 20% of cases are misdiagnosed. Conventional MRI can detect severe atrophy but fails to capture earlier microstructural changes. Advanced microstructural MRI techniques, including non-Gaussian methods like Mean Apparent Propagator (MAP-MRI) and alternative acquisition strategies, show promise in detecting the subtle cellular and molecular changes associated with AD. The first study evaluated microstructural markers of AD pathology across different diffusion metrics. High-resolution imaging of post-mortem human temporal lobe specimens (n = 14) revealed that hippocampal and entorhinal cortex diffusion and restriction metrics strongly correlated with Braak staging, plaque, tangle loads. The second ongoing and complementary study focuses on translating these methods for clinical use by evaluating q-space trajectory imaging (QTI). Imaging a known phantom of liquid crystals, using spherical tensor encoding (STE) and planar tensor encoding (PTE) waveforms achieved acquisition times of ~15 minutes, a significant improvement over traditional multi-shell methods requiring ~48 hours. Preliminary histogram analysis shows promising results in STE’s ability to probe isotropic restriction metrics, resembling return-to-origin probability (RTOP), while PTE required further optimization to mimic propagator anisotropy (PA) distributions. Together, these studies advance the potential of microstructural MRI methods, to detect early and subtle changes in AD pathology. Ongoing developments in QTI techniques aim to bridge the gap for clinical feasibility, advancing diagnostic capabilities.
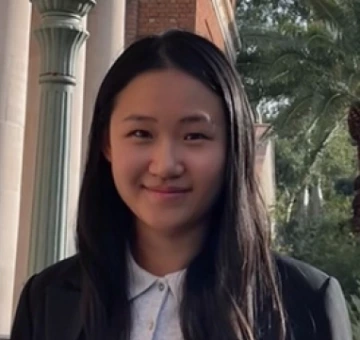
Yisha Tang
Yoon Lab
"AI-Powered Optical Biosensors for Environmental Toxicants and Biomarkers"
Abstract: The detection of environmental toxicants and biomarkers is essential for protecting ecological systems and public health. However, current methodologies often lack the required portability, speed, and precision for effective field applications and point-of-care diagnostics. AI-powered optical biosensors present a transformative approach by combining advanced molecular detection technologies with artificial intelligence, enabling rapid and accurate data analysis and decision-making. These biosensors utilize molecular recognition elements and optical transducers to deliver high sensitivity and specificity across various applications. This research focuses on the development of portable optical biosensor platforms augmented by artificial intelligence frameworks, including computer vision and machine learning algorithms, to process complex image- and video-based datasets. The integration of AI facilitates pattern recognition, contamination level prediction, and scalability through cloud-based data processing. Comprehensive laboratory and field evaluations demonstrate the biosensors’ exceptional sensitivity and specificity in detecting toxicants under diverse environmental conditions and biomarkers in clinical plasma samples. Targets include microRNAs, protein biomarkers, micro/nano plastics, and per- and polyfluoroalkyl substances (PFAS). The findings highlight the transformative potential of AI-powered optical biosensors to advance environmental monitoring, public health diagnostics, and industrial processes. Future research will focus on enhancing detection capabilities through advanced AI models and scaling the platform for broader and more diverse applications.